You signed in with another tab or window. Reload to refresh your session.You signed out in another tab or window. Reload to refresh your session.You switched accounts on another tab or window. Reload to refresh your session.Dismiss alert
weight norm 이 증가하더라. (w-decay 는 어쩌면 좋은 reg 가 아닐 수 있다고 주장 !!)
분석부터 해보자.
loss landscape 를 찍어보면 ViT-SAM 이나 Mixer-SAM 이 확실히 안정적이다.
(regularization term 없이 visualize 한 것으로 보인다. regularization term 을 어떻게 붙이냐에 따라 loss landscape 가 바뀌는 건 어쩔 수 없는 문제라 생각한다. 누가 분석 논문좀 써줬으면...)
NTK kernel은 다음과 같이 정의된다.
이 kernel 값의 eigen value들을 다음과 같다고 할 때,
κ == λ_1 / λ_m 이다.
이 κ 값이 크면 클수록 trainability 가 낮은데, 위 표를 살펴보면 Mixer 의 κ 값이 최악임을 알 수 있다.
hessian λ 값도 크면 클 수록 steep 한 loss landscape 를 가진다고 볼 수 있는데, λ_max 값도 SAM 을 쓰면 줄어 든다. #98 에서도 언급했듯이, resnet 은 이미 global minima 로 잘 가려는 성향이 있어서, SAM 효과가 좀 떨어지는 것으로 보인다.
Augmentation 과 SAM 의 역할은 비슷한 것으로 보인다. (확실하지는 않지만..)
ResNet은 SAM 쓰나 안쓰나 active neuron 수는 비슷하다.
ViT 는 active neuron 자체가 굉장히 적은데, SAM 을 쓰면 활성도가 높아진다.
Mixer 는 active neuron 이 굉장히 많은데, SAM 을 쓰면 줄어든다. (같은 역할을 하는 neuron 들을 없앤다고 저자들은 추측함)
ImageNet 데이터를 분할해서, 좀 더 작은 데이터에서의 경향성을 봤는데, 비슷하더라. (더 큰 실험은?? 궁금한데 ㅠㅠ)
The text was updated successfully, but these errors were encountered:
paper
NTK 도 찾아보게 만들고... 수학공부 다시 시작하게 만들어 준 고마운 논문.
augmentation 이나 pretraining 없는 조건에서는, SAM 이 VIT 나 MLP-Mixer 에 굉장히 잘 적용되고, resnet 을 이기더라.. 하는 논문.
SAM 쓸 때 다음과 같은 현상 발견
분석부터 해보자.
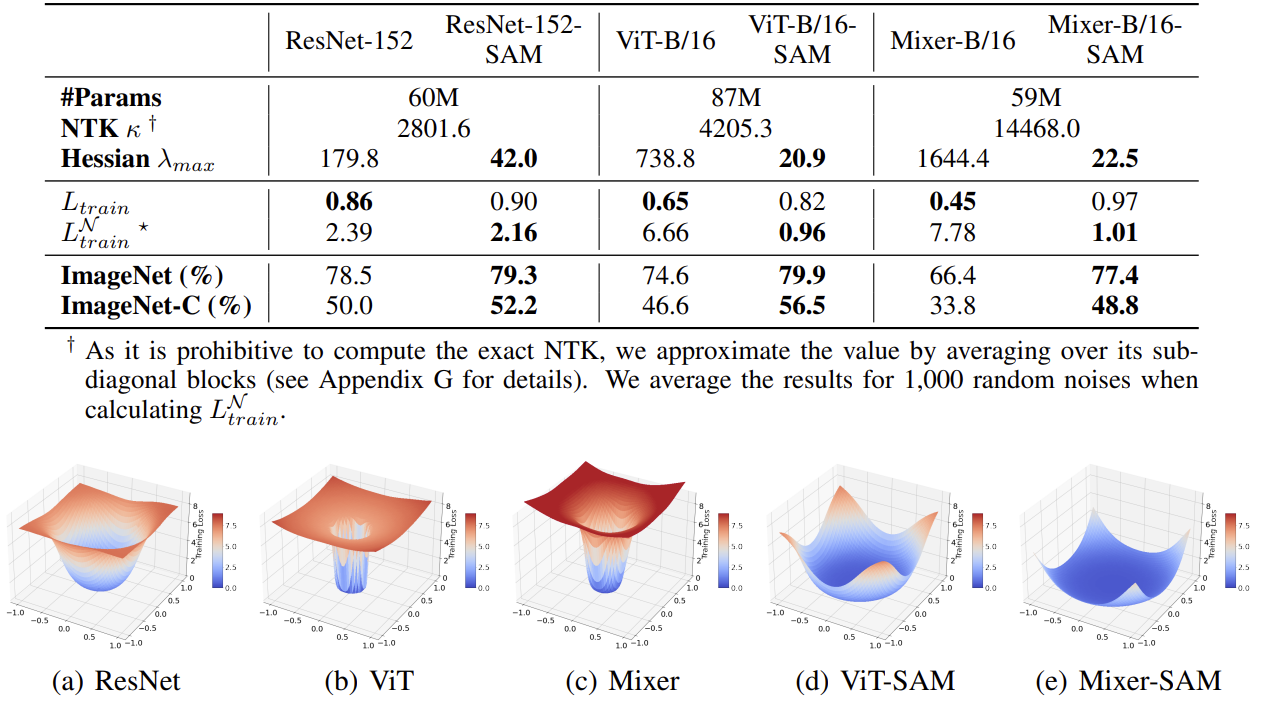
loss landscape 를 찍어보면 ViT-SAM 이나 Mixer-SAM 이 확실히 안정적이다.
(regularization term 없이 visualize 한 것으로 보인다. regularization term 을 어떻게 붙이냐에 따라 loss landscape 가 바뀌는 건 어쩔 수 없는 문제라 생각한다. 누가 분석 논문좀 써줬으면...)
NTK kernel은 다음과 같이 정의된다.

이 kernel 값의 eigen value들을 다음과 같다고 할 때,

κ == λ_1 / λ_m
이다.이 κ 값이 크면 클수록 trainability 가 낮은데, 위 표를 살펴보면 Mixer 의 κ 값이 최악임을 알 수 있다.
hessian λ 값도 크면 클 수록 steep 한 loss landscape 를 가진다고 볼 수 있는데, λ_max 값도 SAM 을 쓰면 줄어 든다.
#98 에서도 언급했듯이, resnet 은 이미 global minima 로 잘 가려는 성향이 있어서, SAM 효과가 좀 떨어지는 것으로 보인다.
Augmentation 과 SAM 의 역할은 비슷한 것으로 보인다. (확실하지는 않지만..)
ResNet은 SAM 쓰나 안쓰나 active neuron 수는 비슷하다.
ViT 는 active neuron 자체가 굉장히 적은데, SAM 을 쓰면 활성도가 높아진다.
Mixer 는 active neuron 이 굉장히 많은데, SAM 을 쓰면 줄어든다. (같은 역할을 하는 neuron 들을 없앤다고 저자들은 추측함)
ImageNet 데이터를 분할해서, 좀 더 작은 데이터에서의 경향성을 봤는데, 비슷하더라. (
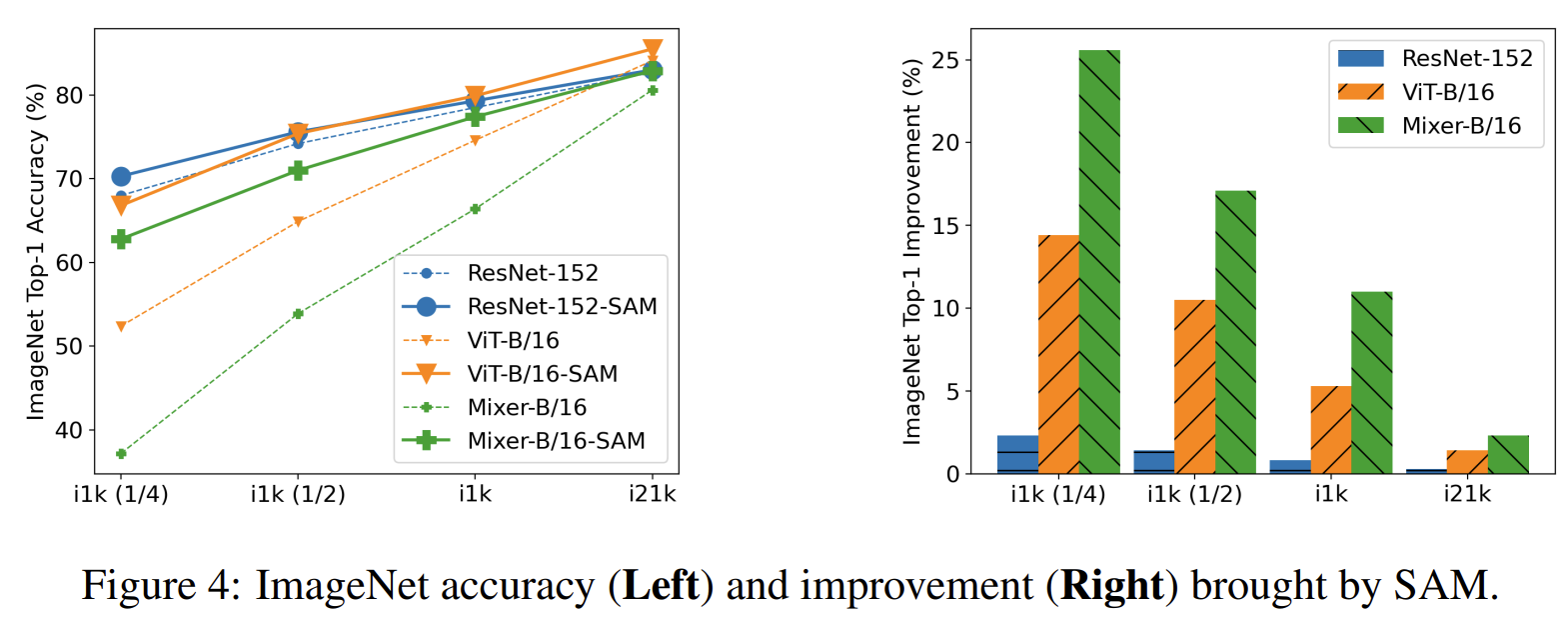
더 큰 실험은?? 궁금한데 ㅠㅠ)The text was updated successfully, but these errors were encountered: