-
Notifications
You must be signed in to change notification settings - Fork 0
/
Copy pathmany_models.Rmd
228 lines (152 loc) · 9.56 KB
/
many_models.Rmd
1
2
3
4
5
6
7
8
9
10
11
12
13
14
15
16
17
18
19
20
21
22
23
24
25
26
27
28
29
30
31
32
33
34
35
36
37
38
39
40
41
42
43
44
45
46
47
48
49
50
51
52
53
54
55
56
57
58
59
60
61
62
63
64
65
66
67
68
69
70
71
72
73
74
75
76
77
78
79
80
81
82
83
84
85
86
87
88
89
90
91
92
93
94
95
96
97
98
99
100
101
102
103
104
105
106
107
108
109
110
111
112
113
114
115
116
117
118
119
120
121
122
123
124
125
126
127
128
129
130
131
132
133
134
135
136
137
138
139
140
141
142
143
144
145
146
147
148
149
150
151
152
153
154
155
156
157
158
159
160
161
162
163
164
165
166
167
168
169
170
171
172
173
174
175
176
177
178
179
180
181
182
183
184
185
186
187
188
189
190
191
192
193
194
195
196
197
198
199
200
201
202
203
204
205
206
207
208
209
210
211
212
213
214
215
216
217
218
219
220
221
222
223
224
225
226
227
---
title: "Fitting many models with `purrr`, `broom`, `dplyr`"
output:
html_notebook:
highlight: tango
number_sections: yes
---
Let's start simple, highlighting some basic tools that we will need later: chiefly, tools from the broom package.
# A tidy workflow for working with one model
## What are tidy dataframes / tidy data?
Hadley (Wickham, 2014) puts this well:
> "tidy datasets provide a standardised way to link the structure of a dataset (its physical layout) with its semantics (its meaning)"
Appearance layout of tidy data:
- Each variable is represented by a single column
- each observation has its own row
- each value has its own cell
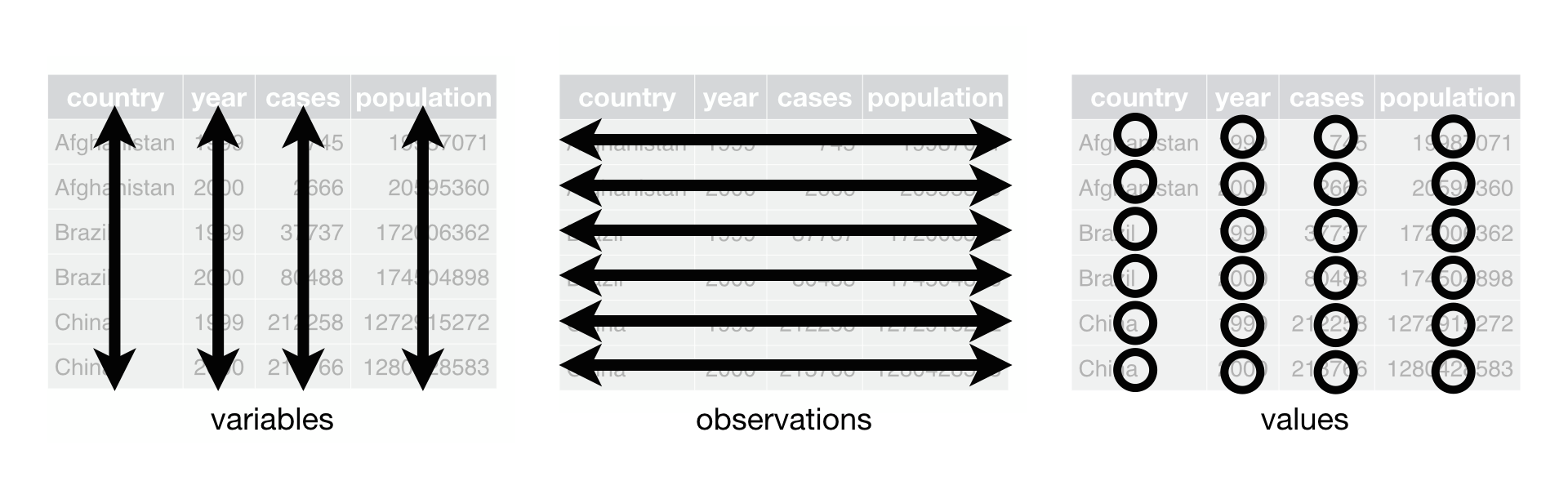
More info: [https://github.com/egouldo/VicBioCon17_data_wrangling/blob/master/modules/06_tidy_data.md](https://github.com/egouldo/VicBioCon17_data_wrangling/blob/master/modules/06_tidy_data.md)
## What's in a model? Sweeping up with broom
Regressions are messy in R...
```{r fit-single-mod}
library(magrittr)
lm_car <- lm(mpg ~ wt + qsec, data = mtcars)
summary(lm_car) # messy output
```
1. Extracting coefficients takes multiple steps `data.frame(coef(summary(lm_car)))`
2. Information is stored in rownames: combining models requires wrangling
3. Column names are annoying: must access with `$“Pr(>|t|)”`, and is converted to `Pr...t..`
4. Information computed in the print method is not stored, e.g. F-stat and p-values
```{r load-broom}
# Enter broom:
library(broom)
```
Broom generates tidy model summaries, turning statistical models into tidy data-frames.
- `broom::tidy` model component-level statistics: coefficient estimates, SE, etc.
- `broom::augment` observation-level, fitted values, residuals etc.
- `broom::glance` - model-level statistics: e.g. ${R}^{2}$ , AIC, deviance etc.
```{r demo-broom-functions}
lm_car %>% broom::tidy() # one observation per model term
lm_car %>% broom::augment() # one observation per observation in the original data, new columns preceded with "."
lm_car %>% broom::glance() # one observation per model
```
Don't know what a pipe (`%>%`) is? Click here: [https://github.com/egouldo/VicBioCon17_data_wrangling/blob/master/modules/05_dplyr-walkthrough.md#writing-sentences-joining-verbs-with-pipes-](https://github.com/egouldo/VicBioCon17_data_wrangling/blob/master/modules/05_dplyr-walkthrough.md#writing-sentences-joining-verbs-with-pipes-)
## Why do we want tidy model summaries?
- The data manipulation and reshaping is done for you, by `broom`. You can focus on understanding your model and your data, rather than on writing code.
- Tidy data works with tidy tools: we can easily visualise broom's output with `ggplot`, e.g. plotting the model in dataspace, generate coefficient plots, survival curves, etc.
- Working with MANY models: tidy model outputs can be easily combined, and compared.
# When do we fit multiple models?
1. Exploring the space of all possible models and their relative merits: E.g. for a given model family, you can explore different forms, for example, for a linear model we could generate all models with main effects.
2. Varying model settings: e.g. systematically alter the tuning parameters to observe the result.
3. Fitting the same model to different datasets: cross-validation, bootstrapping, simulating data, sensitivity analyses, etc.
4. Finding global optima - e.g. when model fitting might not converge to global optimum, you can have a collection of models generated from multiple random starts.
5. Fitting many simple models ot smaller sub-groups of your data rather than a single complex model to the whole dataset
## Computational challenges of fitting many models
We can distill the above tasks into two primary workflows:
1. When you have a single model that you want to repeatedly fit to different sub-sets of the larger data set.
2. When you have a selection of different models you want to fit to the same piece of data repeatedly.
You could solve this computationally by fitting many models using for-loops. Or, when fitting fewer models, you can store your models and their resultant outputs as objects in your global environment.
- Loops: slow, *cumbersome* to write
- Intermediate objects: clutter up your global environment, must keep mental-track of each object
- For either method, you still need to extract the desired model outputs from each fitted model, wrangle them, combine them, perhaps wrangle some more, before you can analyse and/or visualise your many models simultaneously.
But there are a suite of tools out there that get the job done more efficiently (both in terms of computational efficiency, and in terms of the actual code that you write)!
## A workflow for same model, many pieces:
What do we need? (getting around the loop / many objects conundrum)
1. dplyr: create new columns / variables, amend existing ones
2. tidyr: nested data with list-columns
3. purrr: map functions, map nested dataframes to the modeling function or to broom function
```{r load-libs-data}
library(dplyr) # data manipulation
library(tidyr) # For reshaping dataframes, specifically nesting
library(purrr) # For applying functions to nested dataframes
library(ggplot2) # good lookin' plots
spp_mods <- feather::read_feather("./data/grasslands_data")
spp_mods
spp_mods %>%
ggplot(aes(y = percent_cover, x = BG_pc, colour = type)) +
geom_point() + facet_grid(~ type)
spp_mods %>%
ggplot(aes(y = percent_cover, x = E_pc, colour = type)) +
geom_point() + facet_grid(~ type)
spp_mods %>%ggplot(aes(y = percent_cover, x = E_diversity, colour = type)) +
geom_point() + facet_grid(~ type)
```
### nest the data: list-columns
```{r nest}
spp_mods <-
spp_mods %>%
group_by(species, type) %>%
nest()
spp_mods
```
List-columns are great because they keep all related objects together (i.e. in a row). We do not have to keep them manually in sync - the dataframe structure does this for us.
### Define a model, apply it to each species
```{r apply-mod}
species_model <- function(dataframe){
lm(percent_cover ~ BG_pc + E_pc + E_diversity + management, data = dataframe)
}
spp_mods <-
spp_mods %>%
mutate(model = purrr::map(data, species_model))
spp_mods
```
### sweep up with broom
```{r broom}
spp_mods <-
spp_mods %>%
dplyr::mutate(coefs = map(model, broom::tidy),
fitted_vals = map(model, broom::augment),
model_stats = map(model, broom::glance))
spp_mods
```
Viewing tools for nested data frames are not great, yet:
```{r}
spp_mods %>% unnest(coefs)
spp_mods %>% unnest(fitted_vals)
spp_mods %>% unnest(model_stats)
spp_mods$coefs[[1]] # coefs for first spp
```
Plot the coefficients for first 5 spp
```{r plot-coefs}
spp_mods %>% dplyr::slice(1:5) %>%
unnest(coefs) %>%
mutate(lower_CI = estimate - 1.96 * std.error,
upper_CI = estimate + 1.96 * std.error,
significant = ifelse(0 >= lower_CI & 0 <= upper_CI, "no", "yes"),
term = factor(term, levels = term)) %>%
ggplot(aes(y = term, x = estimate, colour = significant)) +
geom_point() +
geom_errorbarh(aes(xmax = lower_CI, xmin = upper_CI), height = 0) +
geom_vline(xintercept = 0, linetype = "dashed", colour = "grey60") +
facet_grid(~species)
```
# What other plots can we make?
```{r}
```
# References:
Robinson, D. (2014). broom: An R Package for Converting Statistical Analysis Objects Into Tidy Data Frames. [https://arxiv.org/pdf/1412.3565v2.pdf](https://arxiv.org/pdf/1412.3565v2.pdf)
Robinson, D., (2015) broom: An R Package to Convert Statistical Models into Tidy Data Frames, Paper presented at UP-STAT2015: Statistical Modelling in the Era of Data Science, SUNY, 4th November 2015, [http://varianceexplained.org/files/broom_presentation.pdf](http://varianceexplained.org/files/broom_presentation.pdf)
Wickham, H. (2014) Tidy data. Journal of Statistical Software. 59 (10). URL: [http://www.jstatsoft.org/v59/i10/paper](http://www.jstatsoft.org/v59/i10/paper)
Wickham, H., Cook, D., Hofmann, H. (2015) Visualizing statistical models: removing the blindfold. Statistical Analysis and Data Mining: The ASA Data Science Journal, 8(4), 203-235, doi: 10.1002/sam.11271
Wickham, H. & Grolemund, G. (2017) R for data Science, Chapter 21 Iteration, O'Reilly, [http://r4ds.had.co.nz/iteration.html#introduction-14](http://r4ds.had.co.nz/iteration.html#introduction-14)
Wickham, H. & Grolemund, G. (2017) R for data Science, Chapter 12 Tidy Data, O'Reilly, [http://r4ds.had.co.nz/tidy-data.html](http://r4ds.had.co.nz/tidy-data.html)
## Some nice examples:
Using `purrr`: one weird trick (data-frames with list columns to make evaluating models easier) [http://ijlyttle.github.io/isugg_purrr/presentation.html#(1)](http://ijlyttle.github.io/isugg_purrr/presentation.html#(1))
Linguistics, TD deletion: [http://jofrhwld.github.io/blog/2016/05/01/many_models.html](http://jofrhwld.github.io/blog/2016/05/01/many_models.html)
K-fold cross validation with `modelr` and `broom` [https://drsimonj.svbtle.com/k-fold-cross-validation-with-modelr-and-broom](https://drsimonj.svbtle.com/k-fold-cross-validation-with-modelr-and-broom)
Tidy bootstrapping with `dplyr` and `broom` [https://cran.r-project.org/web/packages/broom/vignettes/bootstrapping.html](https://cran.r-project.org/web/packages/broom/vignettes/bootstrapping.html)
Modeling gene expression with `broom`: a case study in tidy analysis [http://varianceexplained.org/r/tidy-genomics-broom/](http://varianceexplained.org/r/tidy-genomics-broom/)
# Session
```{r session}
sessionInfo()
```